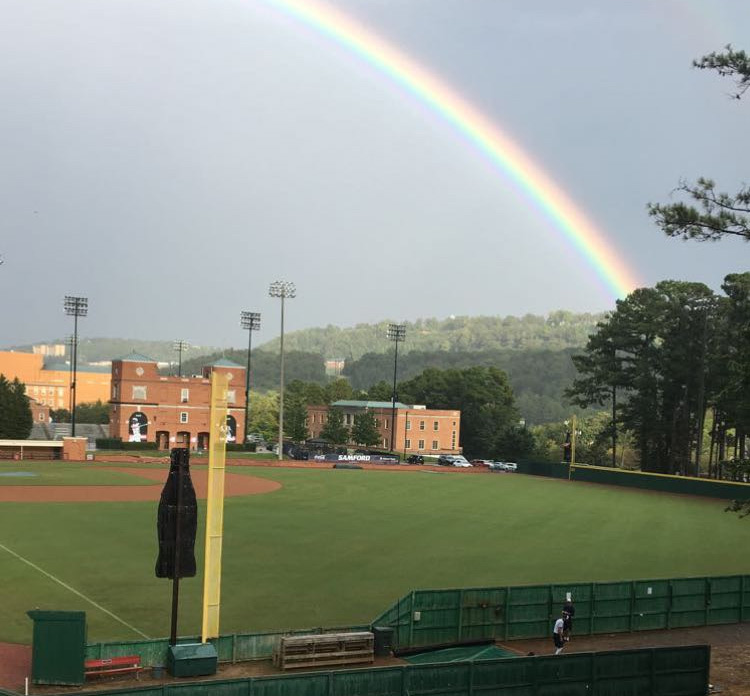
Due to the difficulty and challenges inherent to the sport of baseball, it is in the interest of many inside the sport to examine which factors influence a player’s success within the game. This two-part research series seeks to study geospatial and physical characteristics as they relate to success within baseball. In part one of the series (link), I found that county per-capita median income, marriage percentage, and population were all significantly and positively related with the number of collegiate and professional baseball players produced within a geographic region. In part two, I utilize these same characteristics, along with player height and weight, in an attempt to predict whether the player plays professionally at some point in their career. Having a model that predicts whether a player will play professional baseball at some point in their career can be useful in a number of ways. For example, teams in Major League Baseball (MLB) or independent leagues might utilize the information to focus scouting efforts on amateur players with the highest odds and highest ceiling of achievement. Sports agencies could also utilize the information to target and offer representation to individuals coming from specific environmental factors or physical traits.
Hypotheses
Similar to hypotheses presented in part one of this research, it is expected that county per-capita median income, marriage percentage, and population are all significantly and positively related to a player’s odds of playing professional baseball. Existing research has shown lower income to be a barrier to sport participation (Offord, et al., 1998; Kremarik, 2000; Kokolakakis, et al., 2014). Existing research has also shown household structure to significantly impact sport participation, with children from two-parent households being more likely to participate than those from single-parent households (McMillan, et al., 2016). Larger populations, with a strong countrywide interest such as baseball, are expected to lead to increased levels of participation. More participants should likely herald more professionals from within those regions.
H1: As county per-capita median income increases, so do the odds of a player from that county playing professionally.
H2: As marriage percentage increases, so do the odds of a player from that county playing professionally.
H3: As county population size increases, as do the odds of a player from that county playing at the pro level.
In addition to these geospatial factors, this study examines whether an individual’s physical characteristics have a significant impact on their advancement to the professional level. Larger size, in terms of both height and weight, is expected to significantly impact a baseball player playing at the professional level due to increases in potential power capacity.
H4: As height increases, as do the odds of the player playing professionally.
H5: As weight increases, as do the odds of the player playing professionally.
Analysis
Akin to part one, the dataset contains data on over 38,000 baseball players, each of whom graduated high school from 2009 to 2019 and has gone on to play collegiate or professional baseball. Within the data, the dependent variable of “proball” is coded dichotomously, “1” for playing professional baseball and “0” for not playing any level of professional ball. Also, to account for widespread geographic differences across the United States, county per-capita median income and population were scaled (divided by one thousand). Population is also skewed to the right, so players were placed into six separate and approximately equal population categories. The table below illustrates the logistic regression results.
Seven of the nine independent variables revealed statistical significance, meaning that those seven variables significantly contribute to predicting whether a player plays or has played professional baseball. To help explain the implications of the relationships, as is typical with logistic regression, all estimates are formatted in log odds. The proportional odds ratios for the seven significant variables are given in the table below, along with their corresponding confidence intervals.
While median income shows significant predictive power, the sign is negative, contrary to H1, which means that a unit increase in income decreases the log odds of playing pro ball. Essentially, as county per-capita median income increases, a player’s predicted probability of professional status decreases. This finding is interesting, as in part one of this series it was found that as county per-capita median income increases, as do the predicted number of players produced by that county that play at the collegiate and professional levels. This could mean that while county wealth was found to play a significant role in the production of successful players, it has limited impact in terms of their advancement beyond the collegiate level.
In accordance with H2, increasing county marriage percentage shows to significantly positively impact a player’s log odds of playing professionally. For each one unit increase in marriage percentage, we expect to see an approximate 1% increase in the odds of playing professional baseball.
To test H3, due to the extreme variance in population among counties within the United States, counties were classified into bands of two hundred thousand residents, with the 0–200,000 population range used as the baseline for comparison. Confirming H3, population size significantly impacts the odds of players from that region playing professionally relative to those in the smallest population classification, with the exception of those from the 201,000–400,000 population range. Using 401,000–600,000 population range as an example, these results mean that for players from counties with this population size, we expect to see about 13% higher odds of playing professionally versus the odds of those within the 0–200,000 population bucket. Odds continue to increase in larger markets, as we see it jump to 27% for those in the 600,000–800,000 range, 39% for those 800,000–1,000,000, and an impressive 70% for those in the largest populations (those counties exceeding one million residents).
Physical stature showed mixed results with regard to my hypotheses. Height shows significant predictive power of a player playing professionally, and a positive sign confirms H4. For height, these results indicate that we expect to see an approximate 12% increase in the odds of playing professional baseball for each one-inch increase in height. While weight also indicates a positive relationship with the dependent variable, the estimate is not significant, which is contrary to H5.
The model’s accuracy within the sample’s confusion matrixs 69.7%. This shows that the model correctly predicts whether a player within the dataset has played professionally around 70% of the time. A binary logistic regression model’s AUC, or area under the curve, is an index measure of the model’s predictive accuracy. This model’s AUC is 0.6014, with a perfect predictive model having an AUC of 1. Below is the model’s ROC (Receiver Operating Characteristic) curve, which illustrates the diagnostic ability of the binary classifier system.
Conclusion
County per-capita median income, marriage percentage, and population were all found to impact the odds of predicting whether a ballplayer plays professionally at some point in their career. Similar to part one, these results speak to environmental factors that influence players’ overall achievement in the game over their playing career. Player height was also found to significantly impact the odds of becoming a professional ballplayer, confirming the value of physical stature as a key evaluative metric. These findings can serve professional decision makers from both MLB organizations and player representatives (sports agents) in their future scouting of up-and-coming baseball prospects.
Future analysis might be improved by capturing additional longitudinal data, by both continuing the dataset to see if younger players eventually reach professional baseball and collecting historical data prior to 2009 to see if the trends hold across different generations of players. These updates might also serve to improve the model’s overall predictive power.
References
Kremarik, Frances. 2000. “A family affair: Children’s participation in sports.” Statistics Canada Catalogue 11-008, 20-24.
Kokolakakis, Themis, Lera-Lopez, Fernando & Castellanos, Pablo. May 2014. “Regional differences in sport participation: the case of local authorities in England.” International Journal of Sport Finance Vol. 9, Issue 2.
McMillan, Rachel, McIssac, Michael., Janssen, Ian. 2016. “Family Structure as a Correlate of Organized Sport Participation among Youth”. PLoS One. Vol 11, Issue 2.
Offord, D., E. Lipman & E. Duku. 1998. “Sports, The Arts and Community Programs: Rates and Correlates of Participation.” Ottawa: Human Resources Development Canada. 19.
About the Author
Mathew Bennett is a current MBA student and baseball player at Samford University. After achieving his MBA, Mathew hopes to work in analytics in some capacity within the sports industry.
Twitter: @mattyice_126
LinkedIn